From Bias to Balance: The Critical Role of Diversity in AI
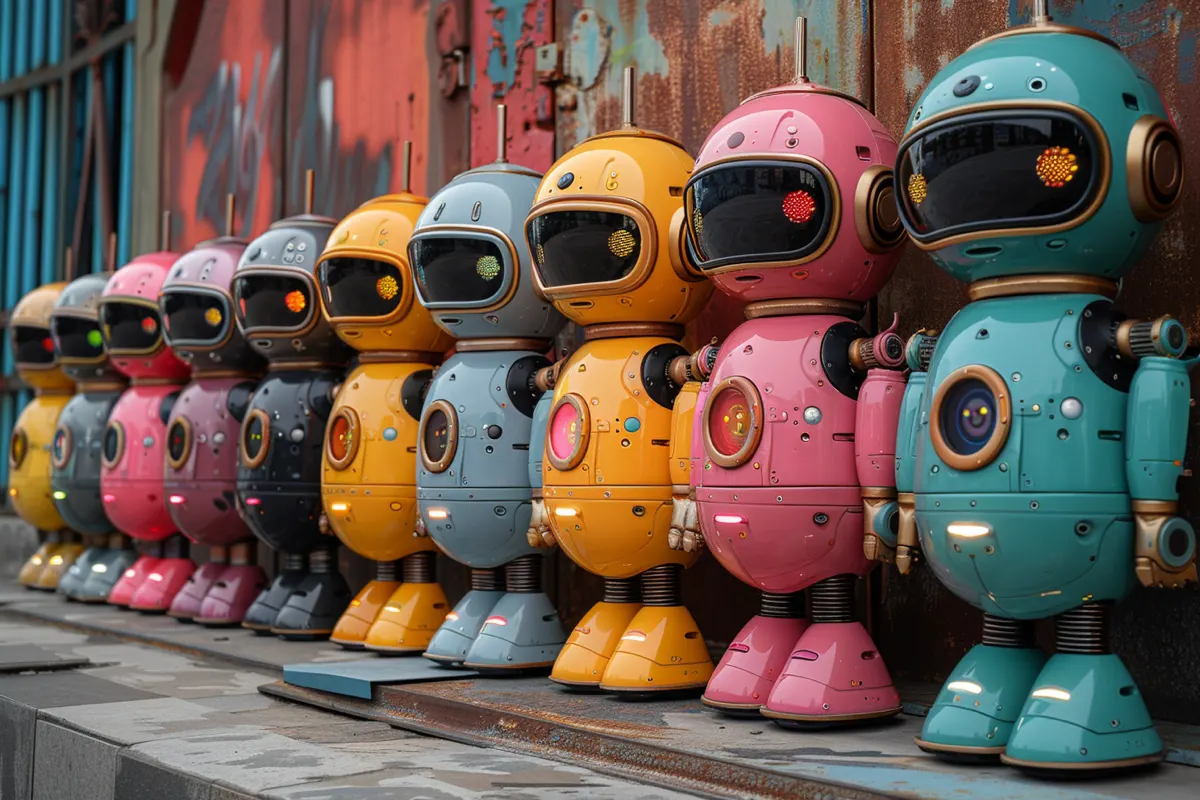
With machine learning and AI solutions becoming an increasingly integral part of our society and everyday lives, one crucial element that needs to be taken into consideration is diversity. Lack of diversity, be it in data, development teams or users, can lead to perpetuating unfair biases and reinforcing societal inequalities. In this context, diversity is not only desirable, it’s imperative. In this article we will not only explain how a model learns bias, but also how it can be used to reinforce it, if left unchecked.
How AI Models Learn
First, let’s start by breaking down how an AI model learns. It’s actually very simple: these kinds of models simply seek to map the available data into a mathematical model. While being trained, the model learns the patterns of the training data, and it recreates those patterns when predicting for new data points. What this means is that the model can only learn and repeat the trends contained within the data it is given. The data is the driving force behind all AI solutions! So where does it come from?
According to Reduced, Reused and Recycled: The Life of a Dataset in Machine Learning Research, around 60% of the datasets used for performance benchmarking in research come from the United States. The image below (credit to the Internet Health Report 2022) illustrates these findings. We can see that entire continents, like Africa and South America, bear almost no representation in benchmarking datasets.
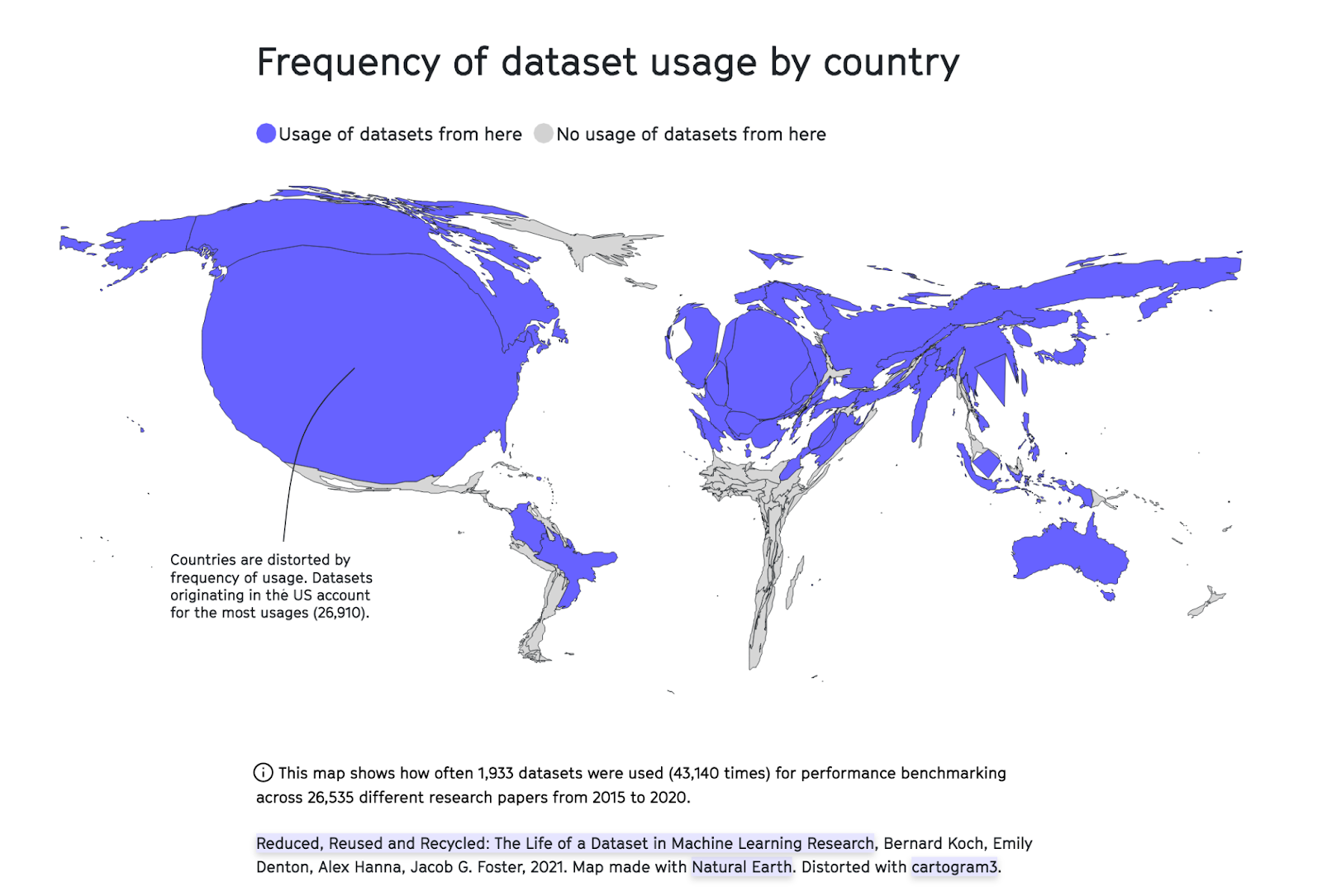
The Source and Bias of Data
At its core, this data bias will mean that AI algorithms aren’t being tested against the breadth of people that will be using them, and there is no assurance that they will work for the other, more diverse, groups. What works for an American population may not be effective for international usage, and this fact can be easily hidden by only using American data to train and, more importantly, evaluate a model.
Another important consideration is that data is made, first and foremost, by people. A lot of the data used for AI development is historical data, not only recorded but also created by humans, and thus is susceptible to human error. Data must be correctly annotated to be used for model training, a task that is often performed by human annotators. Any internal bias in the annotators can easily be replicated in the data and propagated by the final model.
Implications of Data Bias
Let’s get into some real world examples to better understand how data bias may affect real world solutions.
Healthcare: A Case Study in Bias
Healthcare is one of the areas that makes use of AI solutions on what is known as Computer-Assisted Diagnosis (CAD), to lessen the burden on healthcare workers, and allow them to focus on high-priority tasks. However, studies have shown that current datasets do not represent the general population, due to higher income hospitals (which tend to serve mostly White communities) having a larger history of saving electronic health data for their patients. African and Latin-American populations are consistently underrepresented, and therefore more likely to be misdiagnosed by CAD systems. One field where this has been known to happen is dermatology, where certain conditions will have different telltale signs depending on the skin colour of the patient. Often AI models in this field are trained to recognize conditions on white skin, and fail to do so for other skin colours, simply due to the fact that not enough examples of non-white skin were shown to the model. These failings mean that the CAD system does not remove the triage burden from doctors, who still need to check every result carefully, to ensure no patient goes undiagnosed.
The Pitfalls in Targeted Advertising and Recruitment
Another common AI application is to produce targeted advertisements, which has also shown some issues. In 2015, the Washington Post reported that Google’s advertising algorithm tended to show more ads for high-paying executive jobs to men than women. Historically, we can understand where this trend comes from, since women have been pushed out of leadership roles in favour of men in western societies. However, when developing these solutions, cases like this should be identified and the bias should be countered, so that we can ensure equal opportunities for the general population. Similar concerns arise when using AI for recruitment. Even in situations where the gender (or other data, like nationality or ethnicity) of the candidate is omitted to the model, there are other markers (for example, the type of language used in a CV) that may be contributing to unfairness in the final results.
Real-World Consequences of Biassed AI
The data isn’t always the issue, sometimes it is how it’s used. A 2019 study found that an algorithm used to predict health risk for patients was biassed against Black individuals, assigning them lower risk scores when compared to White patients with the same health history. It was found that the developers of the algorithm used annual healthcare spending as a marker of health (higher spending meant higher health risk). Although this association might make sense in some cases, it disregards the fact that, historically, Black people have had more difficulty accessing health care, ending up spending less money than their White counterparts, independent of their actual need. Understanding the societal implications of our data is as important as collecting good quality data when it comes to developing reliable AI algorithms.
Final Thoughts: Combating Bias Through Diversity
The question remains: how do we handle bias in AI solutions? Diversity! Every step of the path that leads to a new and innovative AI solution needs to include a wide breadth of people, from different backgrounds, races, religions and genders. From the data itself, to the team of developers. A diverse and open team will more easily identify the potential downfalls of the system it's creating, and be able to tackle bias before it ever has the opportunity to go out into the world. Another key element is AI governance. Every AI company needs to have policies and guidelines in place to help its employees identify and tackle any fairness, security or privacy issues that can (and will) come up during development.
AI's complexity demands a nuanced approach to safety, where understanding its vulnerabilities becomes central to innovation. Embracing diversity not only enriches AI's development but also ensures its applications are equitable and universally beneficial. As we advance, let's adopt diversity not as a checkbox but as a fundamental principle, pivotal to unlocking AI's potential to serve everyone. This mindset shift is vital for transforming today's challenges into tomorrow's achievements, making AI a tool for universal empowerment.
Let us solve your impossible problem
Speak to one of our industry specialists about how Artificial Intelligence can help solve your impossible problem
