Will AI Kill Music?
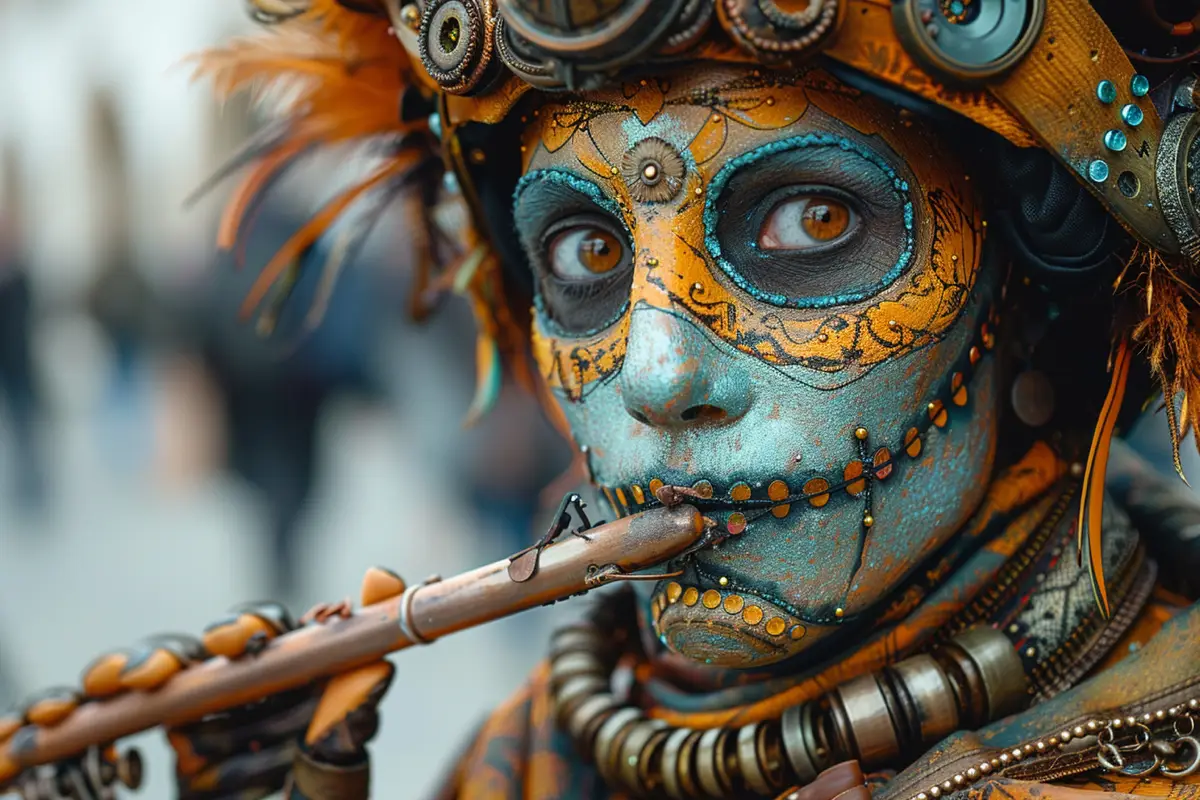
The intersection of Artificial Intelligence (AI) and music is engendering a transformative era in music production, composition, and consumption. With rapid advancements in AI technologies, particularly in Natural Language Processing and machine vision, it is only a matter of time for the next revolution to be in audio. Music AI, leveraging the intrinsic mathematical frameworks of music, is pioneering tools that can identify, deconstruct, and even generate complex melodies and rhythms, signalling a new wave of creativity and innovation.
The Significance of Audio Representations
Understanding the methodologies for audio representation is pivotal for efficient modelling. Two primary forms of audio representation are continuous vectors and spectrograms, both of which allow AI to understand and manipulate music in revolutionary ways.
- Vector Representations. Consider a song as a wave flowing through time. Vector representation captures this wave in a series of numbers, each representing the wave's intensity at different moments. The size of the data generated by audio files is very large, exemplified by a 32 kHz sampling rate requiring a 32,000-sized vector for a mere 1-second clip, which makes dimensionality reduction through convolutions imperative.
- Spectrogram Representations. Transitioning from one-dimensional vectors, spectrograms introduce a two-dimensional matrix representation, akin to visual imagery of audio data, that can be encoded with Convolutional Neural Networks (CNNs), just like images. By dissecting the audio waveform into equal segments and extracting Fourier features from each, a spectrogram is constructed column by column to represent the audio's frequency information over time.
Source Separation and Music Generation: A Dual Perspective
At the forefront of Music AI lie two transformative advancements: source separation and music generation. These developments not only showcase AI's capability to dissect and recreate musical compositions but also hint at a future where AI's role in music transcends mere support to become an integral part of the creative process. Let's dive into the intricacies of these technologies and their potential wider implications.
The Role of Source Separation
Source separation targets the isolation of individual instrument tracks from complete songs, serving to repurpose components for media or artist use, and improving other MusicAI tasks like classification, tagging and data analysis. Technically, it entails breaking down a composite audio wave into its individual sounds (or waves). This is challenging due to the overlapping frequencies in music, but with the appropriate amount of data, it becomes a suitable task for deep learning models.
- DEMUCS. Significant strides have been made with Meta's source separation model “DEMUCS”, whose initial training dataset of a mere 300 tracks, with its individual audio sources, was significantly expanded using data augmentation techniques. Augmentation involves natural modifications of a track (e.g., pitch, speed) as well as generating entirely new compositions by combining disparate elements from multiple tracks, followed by pitch and tempo harmonisation to ensure coherence.
- DEMUCS architecture. This model combines encoding of 1D-wave and 2D-spectrogram data via Wave-U-Net, followed by cross-domain Transformer Encoders optimising a unified latent space, and a Wave-U-Net decoder reconstructing the separate instrument waveforms.
- Reaching beyond traditional limits. A notable development in 2023 from a paper titled “Separate Anything You Describe”, integrated DEMUCS with the CLAP AI model, allowing users to separate sources based on textual descriptions, circumventing the limitations of fixed output categorisations and offering a more versatile tool for music production and sound design.
The Evolution of Music AI: Introducing MusicGen
In June 2023, Meta unveiled MusicGen, surpassing Google’s MusicLM with its use of licensed music, open sourcing, and a novel melody conditioning feature, fostering music creation from both text and melodies.
- Melody Extraction. Instrumental to MusicGen's performance is its method for extracting melodies. Utilising source separation tool DEMUCS, the model removes rhythmic components to isolate the dominant melody of a track, represented through chromagrams.
- Architecture Overview. The architectural design of MusicGen is intricately structured into two primary sections, each dedicated to processing a distinct form of input: textual and auditory. Text is encoded with a T-5 architecture, and the auditory input segment capitalises on a dual-representation approach; the initial representation involves a continuous vector to encapsulate the audio wave, whilst the melody is delineated through a chromagram. The chromagram undergoes encoding through a CNN, and the continuous audio vector is adeptly encoded with EnCodec. EnCodec constitutes an assembly of 1D convolutions paired with Long Short-Term Memory (LSTM) units, a strategic amalgamation designed to preserve the audio's temporal dynamics. Subsequent to this encoding phase, the vector is further compacted and discretized employing Residual Vector Quantization (RVQ). Within the latent space domain, a cross-attention mechanism is employed to synthesise the encoded textual and auditory embeddings. The culmination of this process is realised through an EnCodec decoder, yielding the final audio generation.
- RVQ's Role. RVQ stands as a pivotal compression technique within MusicGen’s architecture, characterised by its ability to transform a continuous vector into a discrete array of indexes. Each index within this array correlates with a specific vector from an array or “CodeBook”, allowing the initial vector to be represented via a combination of vectors from different CodeBooks. The components constituting the CodeBooks are subject to optimization throughout the training phase, ensuring a representation post-RVQ that closely mirrors the original vector. Fundamental to RVQ’s operational premise is its ability to truncate the length of the original vector to the number of CodeBooks employed. Through this mechanism, RVQ effectively reduces data dimensionality, while preserving most of the original information.
- Performance Insights. A noteworthy observation from MusicGen's evaluation indicates a plateau in music quality improvement beyond a model size of 3.3 billion parameters. Despite increasing model size, the generated music did not exhibit further realism or proficiency, though adherence to text prompts continued to enhance.
Final Thoughts
The trajectory of Music AI prompts are a reflection on the balance between technological innovation and human artistry. The potential of AI in music extends beyond automation to become a catalyst for creativity, empowering artists to explore uncharted territories in soundscapes. Yet, the sustainability of careers in music, particularly those reliant on traditional modes of creation, calls for a nuanced understanding of AI's impact.
The evolution of Music AI is not just a testament to technological prowess but an invitation to reimagine the boundaries between the composer and the composed. In fostering a symbiotic relationship between AI and musicians, the future of music looms not as a replacement narrative but as a collaborative symphony of human ingenuity and artificial intelligence.
Let us solve your impossible problem
Speak to one of our industry specialists about how Artificial Intelligence can help solve your impossible problem
