We solve the toughest human problems through the transformative power of Artificial Intelligence

We are the AI partner for business
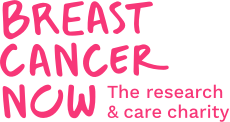
We understand what your business needs to deliver AI projects successfully
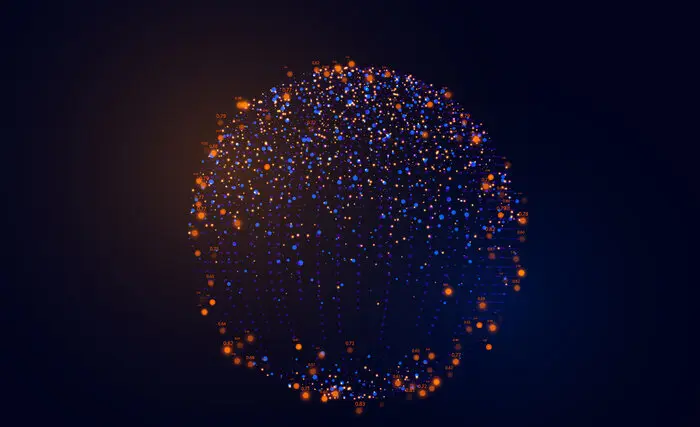
Own the IP
We have built a unique platform called Floatingpoint, which allows you to own your own IP.
We train the model, you own the IP.
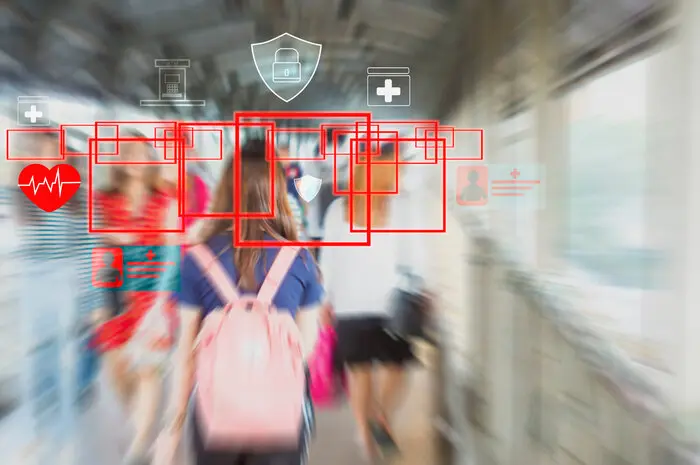
Responsible AI
We work to ISO 27001 infosec standards and follow responsible and ethical AI frameworks.
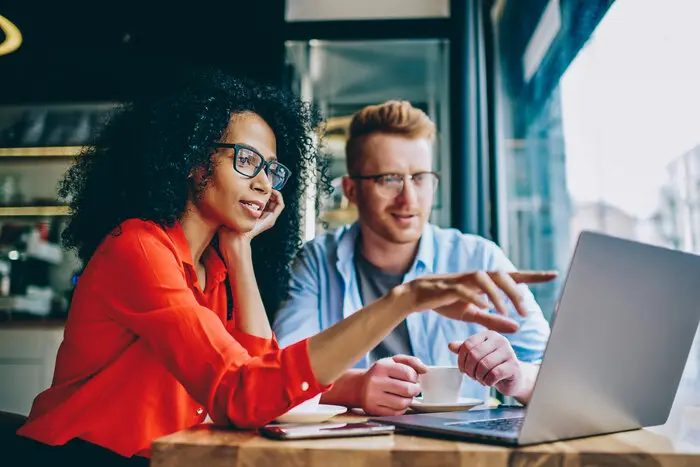
High Perfomance
Our experts build AI systems that can be trusted at scale. We focus on delivering models with over 95% confidence.
The Floating Point Platform
Floating Point is our workflow optimisation platform that allows us to accelerate the delivery of your AI projects
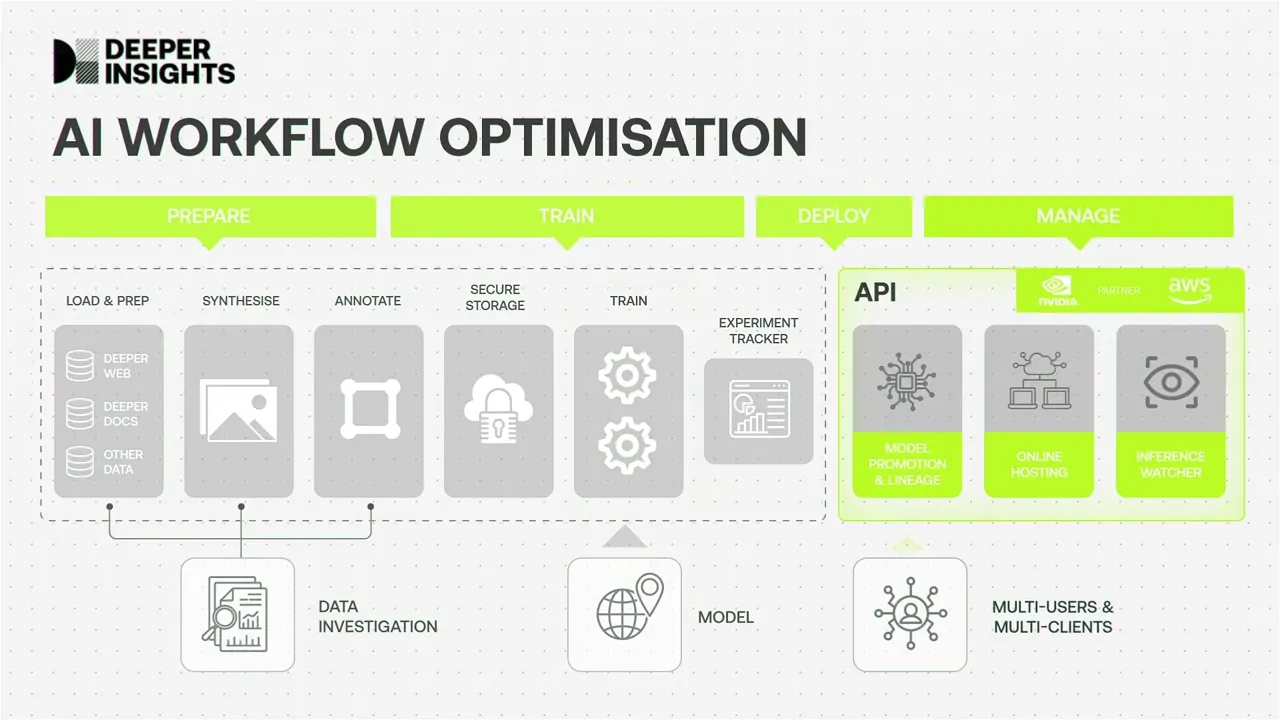
The Floating Point platform brings together and optimises the 5 key stages of delivering an AI project to business
Select
There are 40,000+ Open Source AI models and architectures with unique settings and parameters that can be applied to your business problem.
Floating Point platform enables fast iteration and testing of models to discover the best configuration for solving your specific use case.
The platform allows for repeatable and measurable experiments to determine the optimal solution for your business problem.
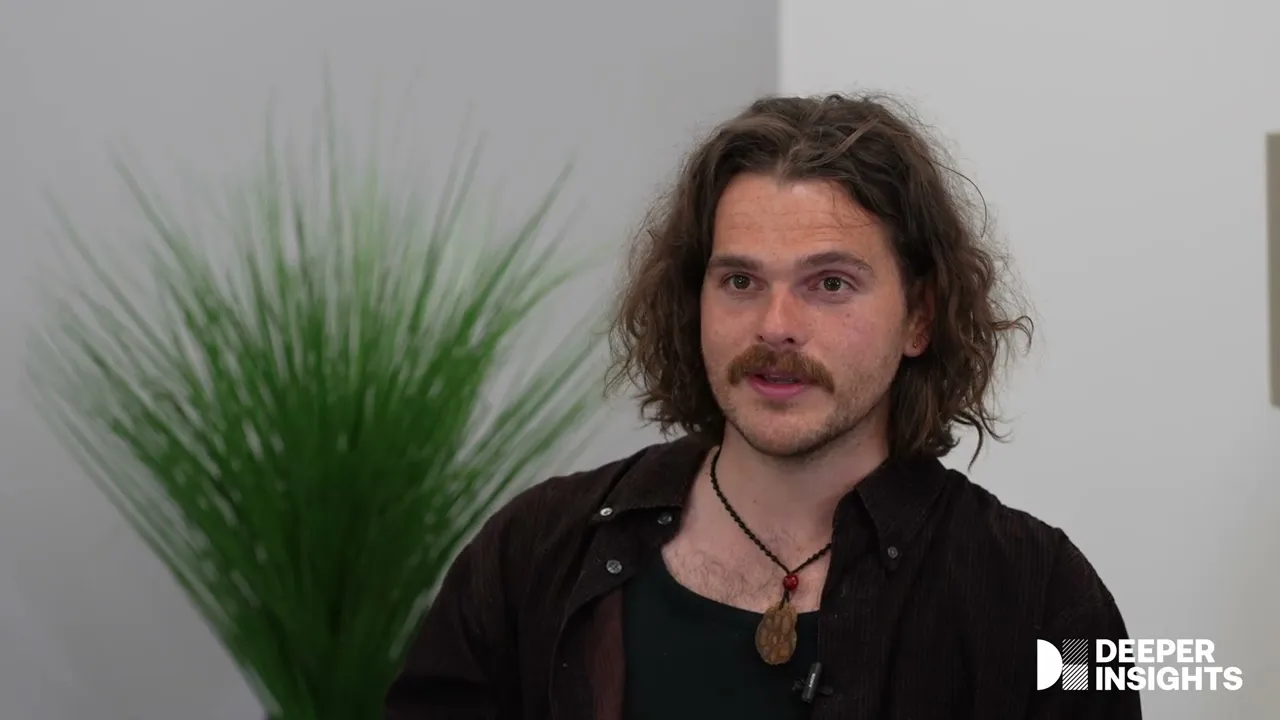
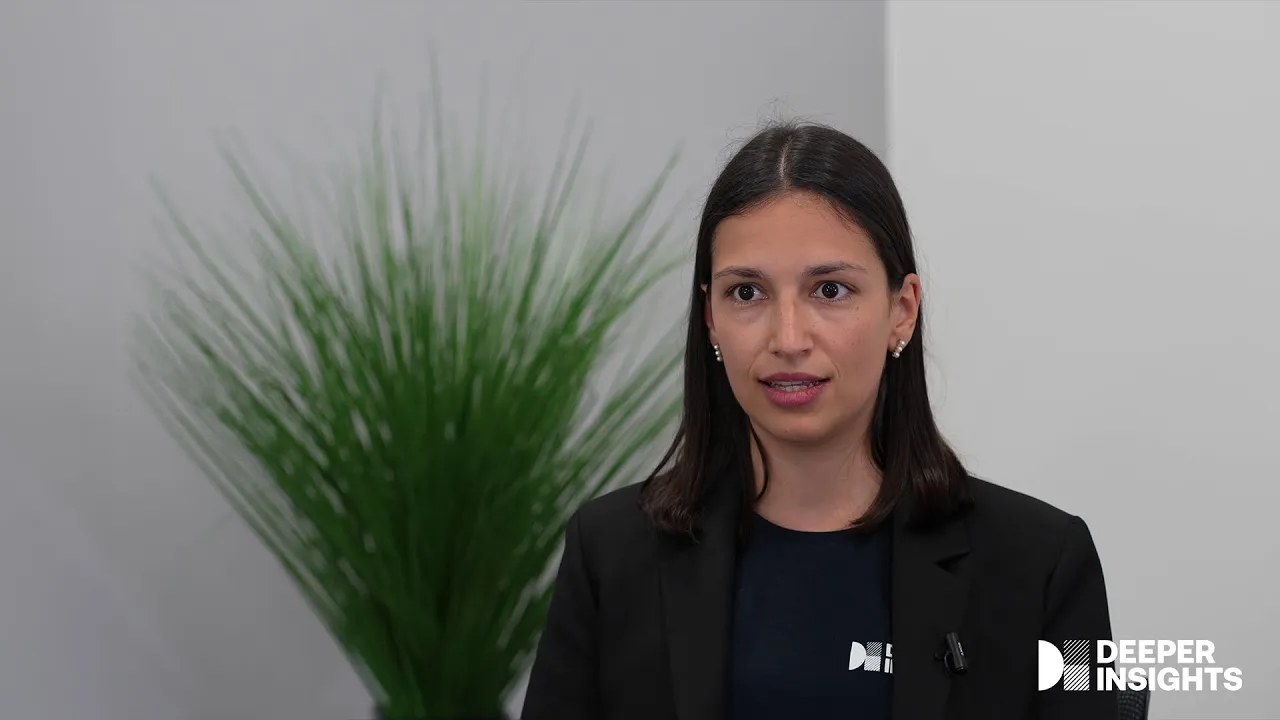
Prepare
During preparation, the Floating Point platform takes in your raw data sets and external data, transforming them into suitable formats for analysis and modeling.
- Data Ingestion: We import the relevant data into the platform in the appropriate format
- Data Processing: We understand the data to be able to put it into the correct format for training the models
- Annotation: If necessary, we annotate the data to create a training data set for supervised learning
Train
The Floating Point platform is both Model-agnostic and Cloud-agnostic, allowing us to choose the optimal infrastructure for your project.
We can monitor the current training process and review past experiments.
This approach enables us to replicate each step of the process, understand model improvement, and foster continuous innovation and iteration in training.
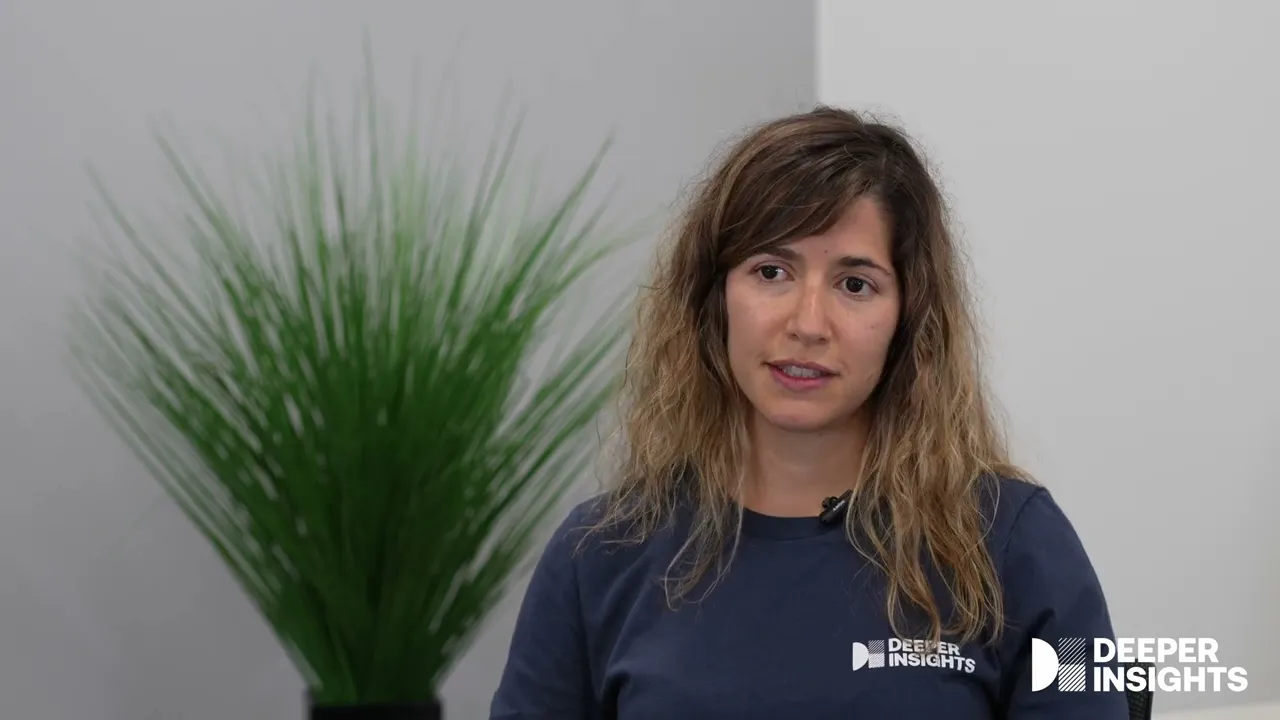
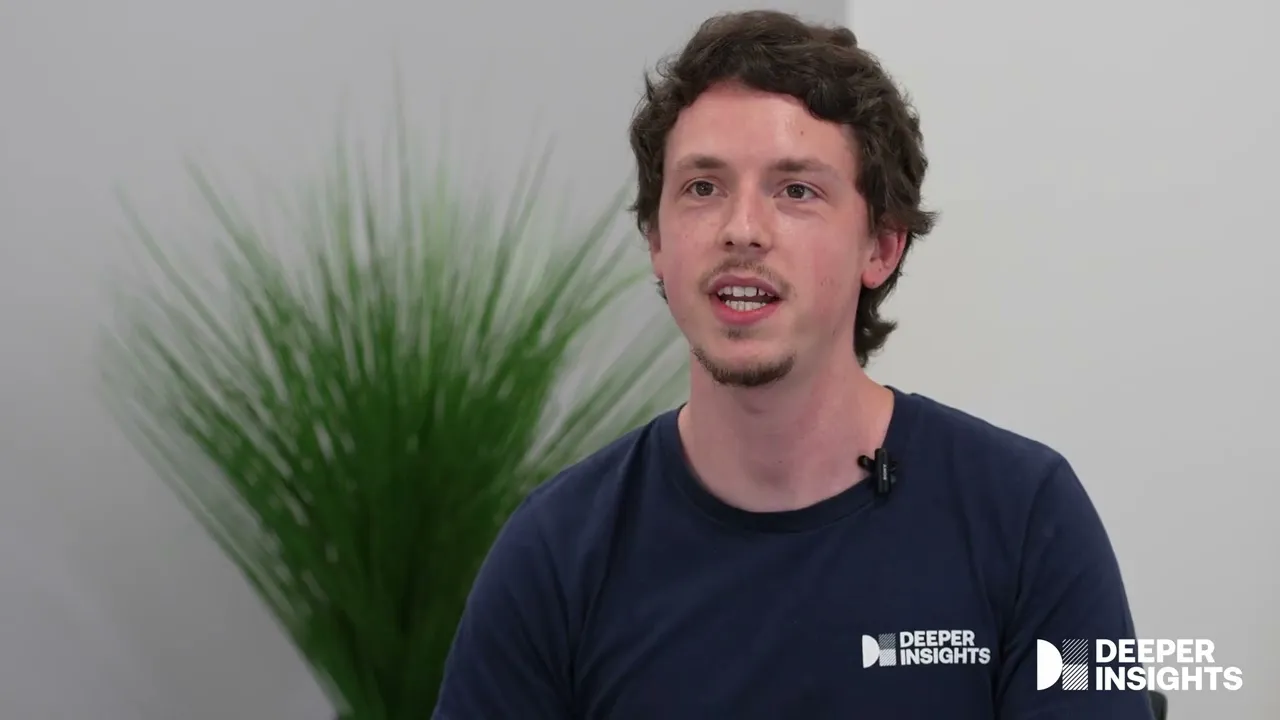
Integrate
The Floating Point platform offers both Cloud and On-premise integration.
Cloud integrations are suitable for companies lacking experienced data engineers, while On-premise integrations cater to clients with specific data privacy and legal requirements.
Once we select the best model from the experiments, based on your agreed success metrics, then we promote it to production and integrate it. The platform is highly scalable, automatically adjusting to meet your business needs in high demand scenarios. This ensures fast and cost-effective model execution in production.
Manage
The Floating Point platform allows us to monitor and manage the AI solution after deployment and while it is in use in the real world. We are able to monitor for data drift over time and to check for any errors in the accuracy of our model over time.
Our dedicated support engineers can monitor, compare and prepare future versions of your model. We are able to monitor for data drift and to check for any errors in the accuracy of our model over time.
The platform enables close monitoring of usage, costs, and scalability aligned with your business needs. It ensures contracted uptime and a high level of service.

How our AI is helping global companies become data driven
Financial Services
Turning billions of data into actionable insights for Financial Analysts
Listen to the AI Paper club podcast
The AI Paper Club podcast is the monthly resource for anyone interested in the world of artificial intelligence. With guests hand-picked by Deeper Insights, each episode dives into the latest academic papers, research, and theories in the field.
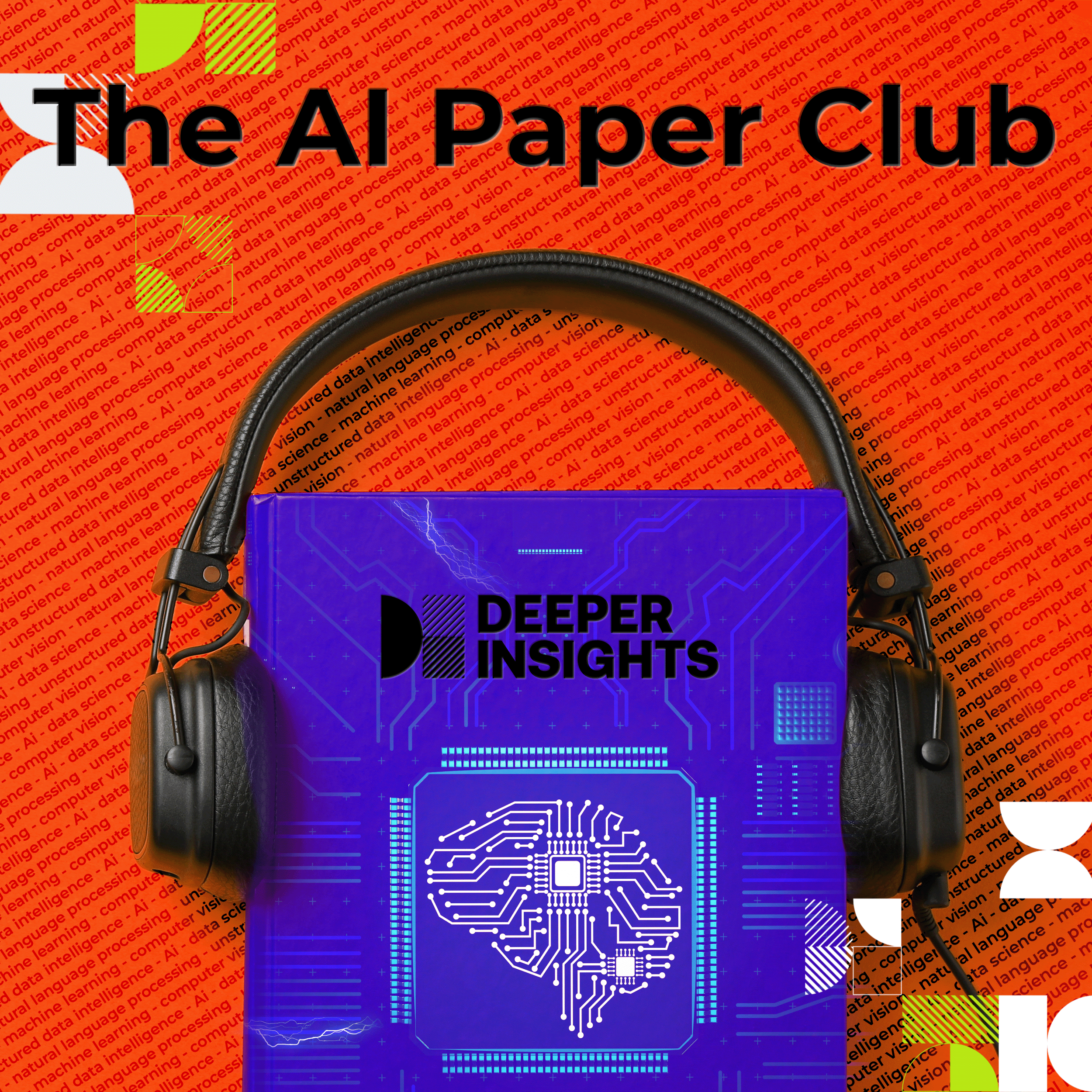
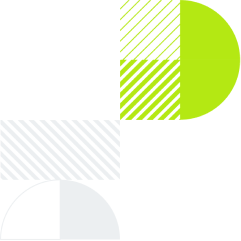

A deeply trusted, expert partner
It's never been done before and will change the category."
Smith & Nephew
GSMA
Let us solve your impossible problem
Speak to one of our industry specialists about how Artificial Intelligence can help solve your impossible problem
